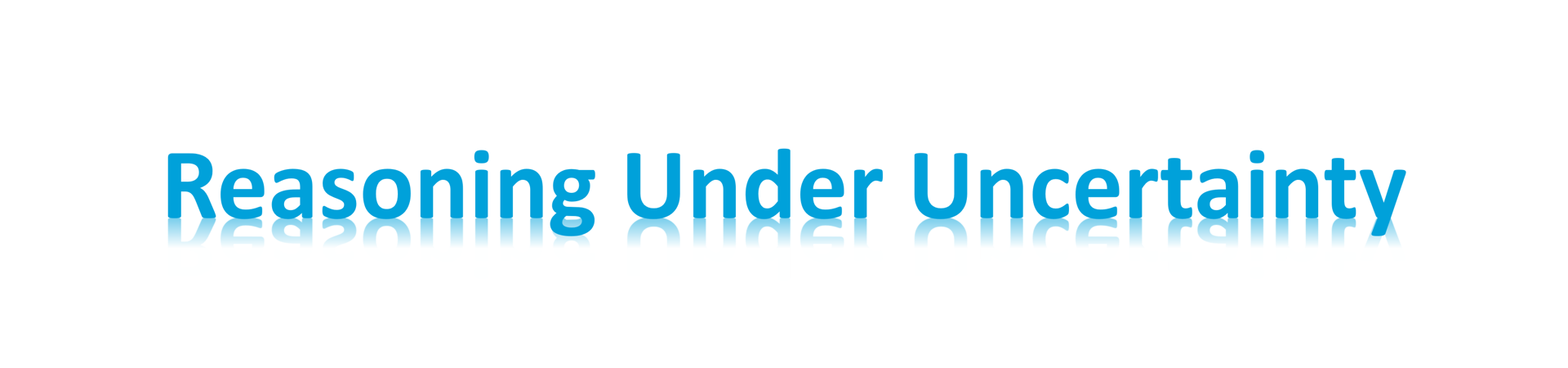
Reasoning under Uncertainity
BackIn contrast to more ‘black box’ models such as (deep) neural networks, probabilistic graphical models (PGMs) such as Bayesian networks consist of variables that explicitly represent meaningful content (symptoms, treatments, observations, signs etc.), that are stochastically and/or causally related to each other. The ability to capture causal relations between stochastic variables and allow for inference to the best explanation of findings, make Bayesian networks a well-established computational formalism for clinical decision support systems. However, in order to construct the next generation of these systems, there are a number of fundamental questions with respect to Bayesian networks that are still to be answered. In computing inferences in the network, statistical information is summarized (marginalized out) that might be important for explicating why a particular advice (and not another one) was reached, and what would need to happen for this advice to change. While work has been done to assess the sensitivity of a particular causal relation for small parameter changes, the question how to use this to report on the trustworthiness of an advice is still open. Finally, changing network structure or parameters on the fly while maintaining continuity and consistency of the statistical model is a fundamental open problem.
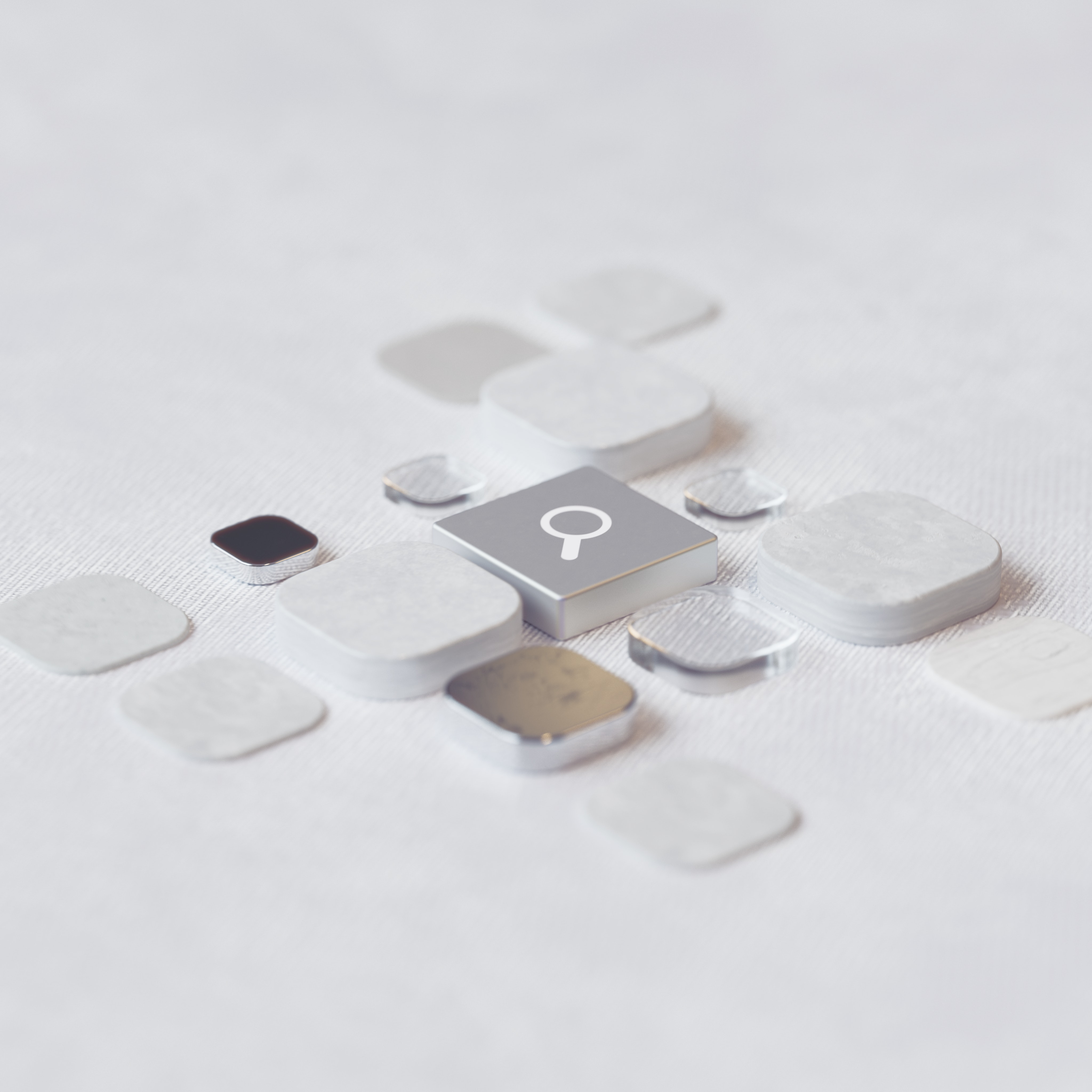
These questions will be addressed in this programme in the following work packages:
1.1 Justification and explanation in Bayesian networks: RU Nijmegen; PI Johan Kwisthout, postdoctoral researcher Natan T’Joens.
1.2 Maintenance and online learning of Bayesian Networks: TU Eindhoven; PI Cassio de Campos; vacancy for postdoctoral researcher; RU Nijmegen; PI Max Hinne; vacancy for postdoctoral researcher.
1.3 Robustness and trustworthy advise; Utrecht University; PI Silja Renooij; Open University; PI Arjen Hommerson; shared postdoctoral researcher Janneke Bolt.